Artificial intelligence is being applied across various business sectors, including agriculture. Alongside AI, other advanced technologies such as the Internet of Things (IoT) and robotics are transforming this sector. This advancement is known as smart farming, which refers to the application of technology to optimize agricultural production.
This concept can sometimes be confused with precision agriculture. However, the latter refers more broadly to the practice of cultivating each part of a property specifically rather than treating it as a uniform area. The foundations for this approach date back to the early 20th century, but it was the development of microcomputers, sensors, and software in the 1980s that made it feasible. Therefore, these two ideas are often associated.
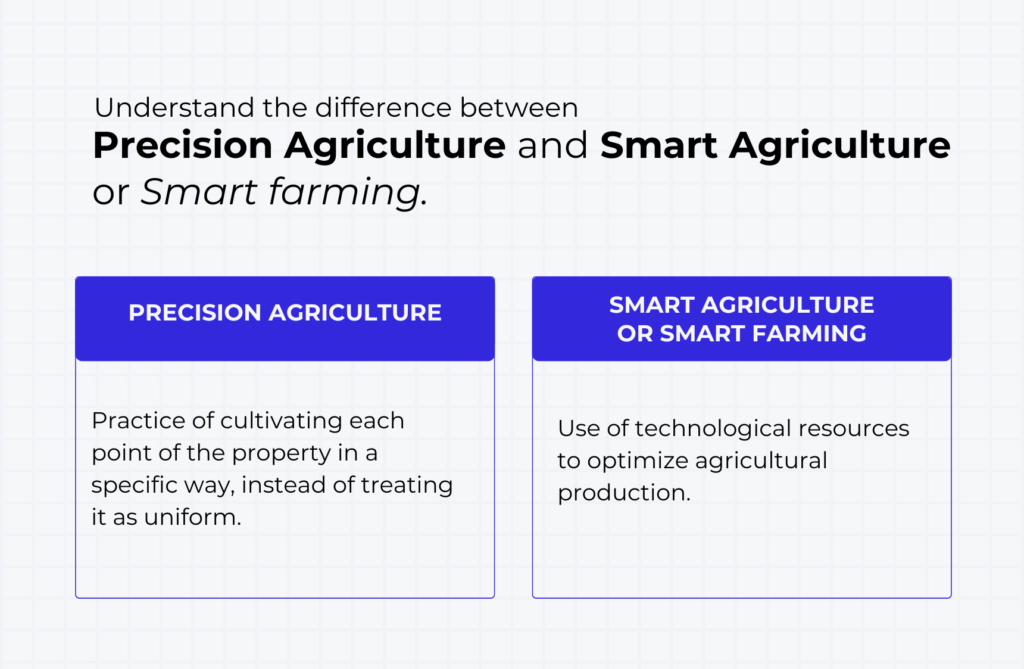
How are large-scale farmers using AI?
Imagine a camera installed in a tomato field that detects the number of tomatoes in an image, analyzes their color, and determines the ideal harvesting time. At the same time, sensors receive this data and automatically adjust the lighting intensity or irrigation frequency. This is how a smart farm operates!
In this example of artificial intelligence in agriculture, computer vision monitors the field while a virtual agent makes decisions based on data—without requiring human intervention. However, there are many other possibilities. Here are some AI use cases to solve common agricultural challenges:
Pest control
AI in agriculture can identify pests in different ways. One approach involves comparing images of the crop with a database of common pests. Additionally, AI can consider other factors, such as the historical presence of pests in that region or local weather conditions.
This enables early identification and elimination of pests, reducing damage and minimizing pesticide use.
Soil monitoring
Continuous soil monitoring allows farmers to cultivate land more precisely, based on its specific needs. AI can analyze soil conditions using sensors installed on the farm or drone images, for example. By assessing characteristics such as moisture, nutrients, and pathogens, AI determines the needs of each section of the land.
As a result, automated irrigation systems can self-regulate, increasing productivity while promoting more sustainable farming with reduced water waste.
Weed control
Computer vision can also detect the presence of weeds, even from a distance. The high precision of AI-powered machines enables targeted weed treatment, whether through mechanical removal or herbicides. This minimizes production losses and optimizes farm maintenance.
What are the challenges of AI in agriculture and how to overcome them?
There are many ready-to-use AI solutions for agriculture, but before implementing this technology, it’s crucial to consider the realities of each farm or agribusiness.
Data collection in the field
Advanced technologies require robust datasets. For AI to monitor a crop, for instance, it must access information about seed types, soil quality, water supply, climate variations, pesticide use, and more. The more accurate the data, the more precise the AI-driven actions. Therefore, it’s essential to provide detailed, up-to-date, and well-organized data in formats such as spreadsheets, images, or text.
Some AI services require an internet connection in the field to receive real-time data, which is not always feasible. However, alternatives exist. Predictive models, for example, analyze historical data and generate future projections, including climate variations that could impact crops.
For machine learning models, which require large datasets for effective training, pre-trained models can be used. These models have already been developed for similar applications and can be adapted through transfer learning—a cost-effective solution.
The key is ensuring that AI relies on trustworthy data.
Business data maturity
Before anything else, a business must be ready for AI-driven technology—both in infrastructure and internal culture. AI needs reliable data, meaning data that undergoes proper collection, processing, and quality analysis. Additionally, AI should be integrated into daily operations. If a system generates valuable insights but is not considered in decision-making due to internal resistance, there will be no real change in results.
Thus, evaluating a company’s data maturity level is essential. If there’s no structured data collection process or if data is incomplete or inconsistent, investing in data engineering solutions might be necessary. This also presents an opportunity to develop a data-driven culture.
Specialized support
Once a business has reached analytical maturity, it must assess its challenges: What is the level of complexity? What resources and time are available? Which systems need integration? Only then should AI solutions be developed.
A specialized company like BIX Tech is a valuable partner in this process, as it can identify opportunities, create tailored solutions, and provide ongoing support.
Make your agricultural production smarter
Now that you know some of the opportunities and challenges of AI in agriculture, you can assess whether implementing this technology in your business is viable. Remember, there are various AI solutions available, and the most important factor is choosing one that makes sense for your specific needs.