Data science (DS) and artificial intelligence (AI) are revolutionizing industries by enabling companies to harness the power of data to make smarter decisions, optimize processes, and predict future outcomes. At the forefront of this transformation is generative AI, an innovative branch of AI that is reshaping how we approach problem-solving, creativity, and automation.
While data science traditionally focuses on analyzing existing datasets to find patterns and generate insights, generative AI takes this a step further by producing entirely new content, whether it’s text, images, designs, or even synthetic data. The potential applications of this technology are vast, pushing the boundaries of how we interact with data and what we can achieve through AI.
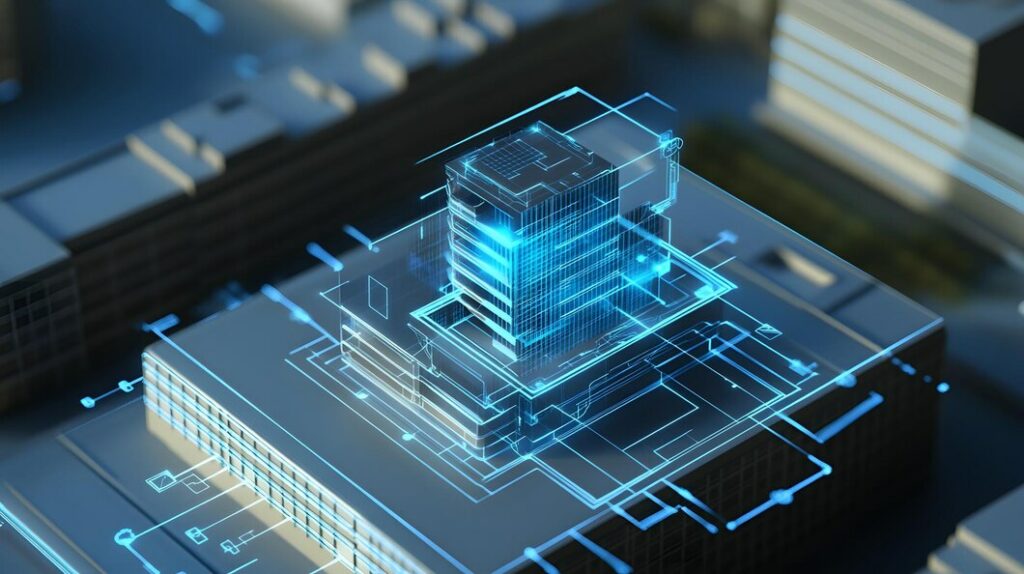
What is Generative AI?
Generative AI refers to a subset of artificial intelligence that leverages algorithms to create new content or data based on input data. The most popular methods within this domain include Generative Adversarial Networks (GANs), which pit two neural networks against each other to generate realistic outputs, and transformer models, such as GPT (Generative Pre-trained Transformer), which are particularly effective for tasks like natural language generation.
Rather than simply analyzing or classifying data, generative AI uses its understanding of patterns within the data to generate new, often novel outputs. This has led to significant breakthroughs across multiple industries, from art and entertainment to healthcare and engineering.
Applications of Generative AI in Data Science
1) Content Creation and Personalization
One of the most exciting applications of generative AI lies in automated content creation. Businesses can now leverage this technology to produce articles, social media posts, marketing copy, and even multimedia content like images and videos. What sets generative AI apart is its ability to personalize content based on user behavior and preferences, making marketing campaigns more effective and engagement rates higher.
For example, AI-driven tools can generate personalized product recommendations, website content, or email newsletters, all tailored to individual customers. This enhances customer experiences by delivering more relevant and timely information.
2) Data Augmentation for Model Training
In the world of data science, having access to large and diverse datasets is critical for training accurate models. However, collecting and labeling data can often be a costly and time-consuming process. Generative AI addresses this issue through data augmentation—the process of generating synthetic data to supplement real-world datasets.
This is particularly useful in fields like healthcare, where patient data can be scarce or restricted due to privacy concerns. By generating synthetic patient records, medical researchers can create larger datasets for training machine learning models, improving the accuracy of diagnostic tools or treatment plans without compromising privacy.
3) Enhancing Predictive Modeling and Simulations
Predictive analytics is a cornerstone of data science, and generative AI is taking it to the next level. Traditional AI models analyze historical data to make forecasts, but generative models can create detailed simulations of potential future outcomes. For example, in financial markets, generative AI can simulate a wide range of economic conditions, helping investors or businesses better understand risks and opportunities.
These simulations enable companies to make more informed decisions about resource allocation, inventory management, and long-term strategies by exploring “what-if” scenarios in a controlled environment.
4) Creative Problem Solving and Innovation
Generative AI is also a valuable tool for creative problem-solving. Its ability to generate alternative designs or solutions allows companies to explore ideas that humans may not consider, leading to more innovative outcomes. In fields like architecture, product design, and engineering, generative AI algorithms are helping professionals come up with optimized solutions that balance multiple factors such as cost, performance, and sustainability.
For instance, generative design in engineering uses AI to suggest thousands of potential design iterations for products, allowing teams to select the best option based on predefined criteria. This technology is not just limited to physical products—it’s also being applied in software development to automate the coding process and enhance program performance.
Challenges and Ethical Considerations
While generative AI presents incredible opportunities, it also raises important challenges and ethical concerns. One of the most pressing issues is the potential for bias in AI-generated outputs. Since generative AI models learn from existing datasets, any biases present in the original data can be perpetuated or even amplified in the generated content. This can lead to unintended consequences, especially in sensitive applications like hiring, healthcare, or legal decisions.
Another challenge is the ownership and originality of AI-generated content. As generative AI becomes more sophisticated, questions arise about who holds the intellectual property rights to AI-generated works. This is particularly relevant in creative industries where human artistry and authorship have traditionally been key.
Moreover, the rise of AI-generated deepfakes— hyper-realistic but entirely fake videos or images— has introduced new risks in terms of misinformation and digital security. As generative AI becomes more accessible, companies and governments must work to ensure ethical guidelines are in place to prevent misuse.
The Future of Generative AI in Data Science
Despite these challenges, the future of generative AI in data science is bright. As models continue to improve, we can expect even more advanced applications, including:
- AI-Generated Research: Generative AI could assist researchers by rapidly analyzing complex datasets, identifying patterns, and even proposing hypotheses, accelerating scientific discovery.
- Automated Software Development: AI could eventually write and optimize code autonomously, reducing development time and improving software performance.
- Virtual Assistants: As generative AI enhances language understanding, virtual assistants will become more intuitive, providing businesses with new ways to interact with customers and manage operations.
As we stand at the intersection of AI and data science, generative AI will undoubtedly play a critical role in transforming how businesses operate, create, and innovate.
Wrapping things up
Generative AI is reshaping the landscape of data science by unlocking new possibilities for creativity, automation, and predictive modeling. From content creation to data augmentation and simulations, this technology is poised to revolutionize industries and redefine what’s possible with data.
Interested in learning how generative AI can elevate your business? Click the banner below and talk to one of our specialists to discover tailored solutions that fit your needs and industry!