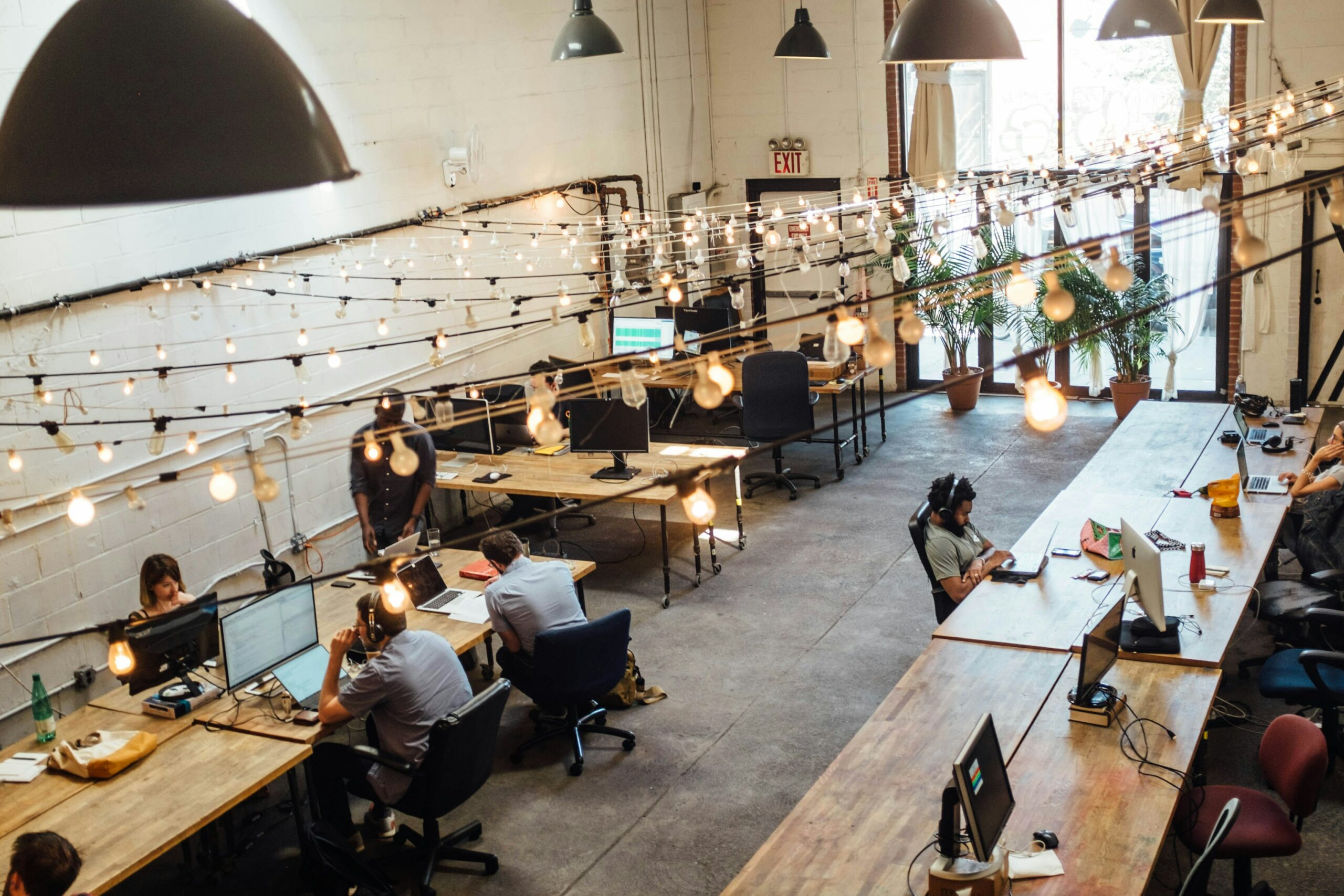
In recent years, the digital universe has witnessed an unprecedented explosion in the amount of data generated, driven by Big Data, the proliferation of the internet, and social networks. This exponential growth has presented significant challenges for traditional data manipulation tools, leading to the need for innovation and evolution. This is where Big Data comes in, a powerful tool designed to handle massive volumes of information efficiently and effectively. Before we explore current trends and the future of this technology, it is crucial to understand its roots and fundamental characteristics.
Before discussing the direction this tool is taking, a relevant question is:
With the advent of the internet, and later social networks, the volume of data in recent years has significantly increased. This rendered the tools previously used to manipulate this data limited and insufficient. Big Data, therefore, addresses the demand that traditional databases are unable to meet, no matter how advanced and extensive they may be.
Formally, it is a set of techniques and computational resources based on programming and algorithms capable of processing and analyzing an extremely large volume of data.
Data related to Big Data comes from various sources, classified into two categories:
Structured Sources: These are data stored in traditional databases, organized in tables, manifesting in the form of numbers or characters. These data have a fixed schema, well-defined format, and simplicity in relating information.
Unstructured Sources: These are mixed data from various sources such as video, text, audio, images, etc. These data lack a predefined type or regular structure and have a certain ease of alteration. This type of data makes up the majority of Big Data.
Traditional tools can handle structured data but struggle with unstructured data. When we mix these two types of data and find correlations between them, we have a massive volume of information.
Volume: Refers to the enormous amount of data involved, as the name suggests. According to a study by IDC, the volume of digital content is doubling every two years. In 2013, there were about 4.4 trillion gigabytes on the planet, and this number is expected to reach 44 trillion gigabytes by 2020.
Velocity: Data is generated very rapidly. The need for real-time data generation, as in the case of sensor usage, digital inputs, and passwords, is becoming increasingly apparent. Velocity is defined by how quickly data is retrieved, stored, and recovered.
Variety: A large volume of data precedes a great variety of data. Thus, Big Data includes not only common transactional data but also data from web pages, search indexes, forums, social media, various sensor data, IoT, and so on.
Veracity: This aspect refers to the reliability of the data. For effective data manipulation in Big Data, the data must be consistent, realistic, and derived from facts, not opinions.
Value: Value is about finding an application in Big Data that allows increasing the company’s revenue in some way. Identifying new opportunities, saving costs, improving product quality. Regardless of the best application for a company, it only makes sense to use it if the data adds value to the organization.
The uses of Big Data vary, and companies from various sectors have benefited from this tool. For example, Netflix and YouTube can recommend movies and videos that fit the user’s profile through the use of databases. Along the same lines, Spotify is also a great example.
Other examples include monitoring social networks, web analytics (e-commerce sites), analyzing financial data to prevent fraud, medical data analysis, personalized and targeted advertising, real-time traffic information, etc.
As we can see, Big Data is not just a common topic in the lives of people working with technology but a reality that is present and influencing all of us.
To manipulate different types of data and find efficient applications for them, Big Data relies on a vast array of technologies, such as distributed file systems, massive parallel processing, cloud computing, data mining grids, high-speed networks, scalable storage systems, specific algorithms, artificial intelligence, among others.
What is the difference between Business Intelligence and Big Data?
Business Intelligence aims to analyze a business’s database to optimize decision-making, generating efficient strategies that benefit the company in some way.
In a visual sense, data in BI solutions is accessed through interactive dashboards and dynamic tables. These tools indicate the performance of the company and its team, providing information to improve business development.
BI solutions have a Data Warehouse, which is a database modeled for queries. The data warehouse stores detailed business data and, through histories, can create and organize reports that will help the company make informed decisions.
In this sense, the major difference between BI and Big Data is that BI has ready-to-use data and facilitates access to information. While BI uses the past to interpret present-day information in the company and thus indicate the best business strategies, Big Data seeks and structures new information that can be used later to optimize and enrich the BI process.
Big Data and Business Intelligence are different but extremely complementary things. In many cases, these two tools work together within an organization to optimize data usage and improve analyses.
The differentiating factor of Business Intelligence is working with “ready-made” data. BI quickly analyzes this information, providing trends, ideas, and guidelines to its managers. On the other hand, Big Data focuses on processing generalized data for information retrieval. Its differentiating factor is the ability to handle a large volume of data at high speed.
Based on the transactional data produced by organizational operations, BI can maintain the data history of companies and provide reports, dashboards, and charts. Big Data, however, is simply the data – just data with an extensive diversity of data types and sources and in large volumes.
Data is the key element of Business Intelligence. Whether “big” or not, data is always the raw material upon which dashboards and analysis reports will be created. Thus, we can say that Big Data functions as an element of the architecture of Business Intelligence.
Due to its ability to capture information from non-structured data sources, Big Data can add more value and accuracy to Business Intelligence. Through the alliance between collecting data from open sources on the internet and extracting data from internal processes, there is the possibility of creating an intelligence system that can point out what is happening inside and outside the company, generating holistic reports that can assess the best guidelines to be taken.
In this way, the use of Big Data is naturally integrating more and more with the use of Business Intelligence. The use of Big Data and BI together allows companies to gain a competitive advantage and achieve more efficient results. The improvement of using Big Data and BI together is most keenly felt in data-driven companies that make use of Advanced Analytics (using data to find patterns, predict, and create scenarios).
A study conducted by CompTIA (Big Data Insights and Opportunities) in the USA showed that 60% of companies depend on data to make market decisions. Furthermore, the study also indicates that 72% of companies that started using tools like Big Data and BI found that the results exceeded their expectations.
Has your company not adopted this type of technology yet? Rely on BIX!
Didn’t find what you were looking for?
Get in touch with our team (786) 558-1665 or send an email to [email protected]. If you prefer, click here and schedule an appointment.